Scaling AI responsibly: Why governance matters for IT leaders
AI is changing how businesses operate. But without the right guardrails, it can quickly become a liability. For IT leaders, AI governance is now essential. It's the key to ensuring that AI systems are secure, compliant, and aligned with business goals.
Why Governance Is Critical
AI systems are different from traditional IT. They can be unpredictable, opaque, and heavily reliant on data quality. Without proper oversight, they pose real risks - from biased decisions to regulatory violations. AI governance helps organizations navigate these risks by putting clear processes and controls in place for how AI is developed, deployed, and maintained.
It’s not just about compliance. Governance also ensures that AI investments generate business value, by aligning technical implementation with strategic priorities and protecting sensitive data and intellectual property along the way.
Key Components of Effective AI Governance
🛡️ Risk Management
AI introduces unique challenges - like opaque decision-making and unpredictable model behavior. Technical teams need real-time monitoring that can detect anomalies, flag compliance issues, and trigger mitigation protocols automatically.
🔐 Data and Access Controls
AI is only as trustworthy as its data. Governance frameworks must include:
- Data quality and privacy checks
- Role-based access controls for development and deployment
- Secure pipelines that comply with GDPR and other regulations
📊 Compliance and Reporting
With regulations like the EU AI Act introducing tiered risk categories, technical leaders must be able to:
- Classify AI systems based on risk level
- Apply appropriate controls
- Generate audit-ready compliance reports
🧩 Integration and Scalability
Governance shouldn’t slow you down. It should integrate seamlessly with:
- Your CI/CD pipeline
- Security infrastructure
- Cloud and data platforms
Hybrid governance models (combining centralized oversight with decentralized flexibility) often work best for scaling across large enterprises.
Measuring What Matters
To demonstrate the value of governance, technical teams should track both performance and impact. Compliance rates, speed of risk mitigation, and the effectiveness of access controls all show how well governance is working. At the same time, metrics like AI-driven productivity gains or cost savings help connect governance to business outcomes.
User adoption is a telling indicator as well. If governance frameworks are too rigid, they’ll be bypassed. But if they’re designed for usability, teams will integrate them into their workflows - making governance part of how AI is built, not a barrier to innovation.
How to Get Started
Implementing AI governance doesn’t have to be overwhelming. Start with a phased approach:
- Foundation: Monitor existing AI systems and define key policies.
- Expansion: Introduce automated compliance tools and integrate with enterprise systems.
- Optimization: Automate repetitive tasks, track KPIs, and evolve governance as regulations and AI use cases mature.
Ready to Take the Next Step?
📅 Schedule a free AI Governance Assessment here
Our experts will evaluate your current setup and recommend a technical roadmap tailored to your regulatory environment, risk profile, and maturity level.
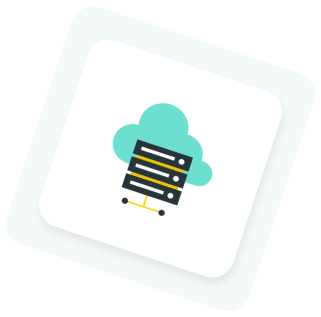